
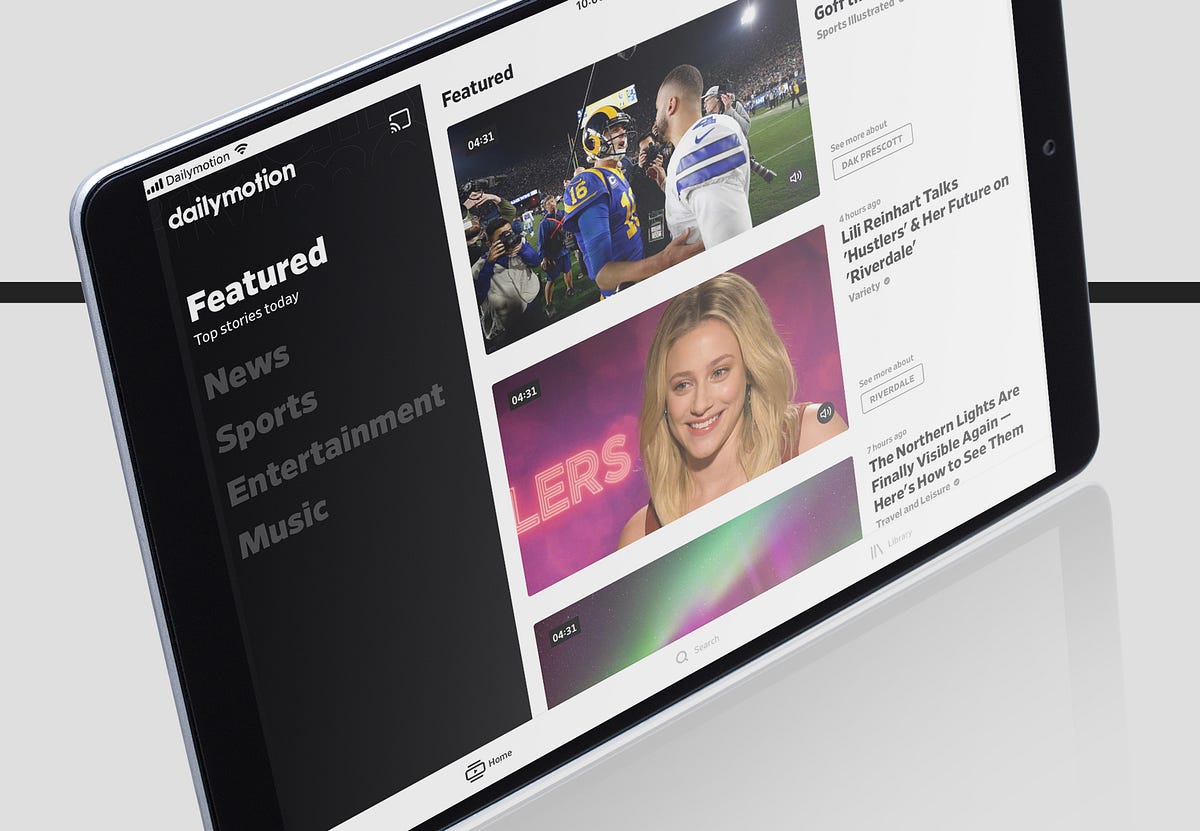
- Airflow kubernetes pod operator example install#
- Airflow kubernetes pod operator example software#
- Airflow kubernetes pod operator example free#
RUN apt-get update & apt-get install google-cloud-sdk -y RUN curl | apt-key -keyring /usr/share/keyrings/ add. RUN apt-get install apt-transport-https ca-certificates gnupg curl -y RUN echo "deb cloud-sdk main" | tee -a /etc/apt//google-cloud-sdk.list I had issues with getting gcloud and python installed in alpine.
Airflow kubernetes pod operator example free#
A yaml file to create a docker image of your repo in the Artifact repositoryįeel free to try alpine instead of ubuntu.To enable this, we created a base_dbt_docker repo with the following files: This means that there is a central image for updating versions and also compilation time for docker image using this dbt docker image is much faster. We decided to use a separate docker image that contains all the “installs” to execute a dbt command. Use the KubernetesPodOperator in your DAG.Create a Docker image Artifact of your dbt repo.Create a base Docker image Artifact of containing dbt, python, git and gcloud.However, we have already been doing this as part of support. And we need to scale it up or scale it out if it is needed. This option will require monitoring on the Composer resource usage. Since the dbt models are docker containerised, dependency conflict issue will not be a problem.Īs the KubernetesPodOperator will automatically spin up the pods to run the docker image, we do not need to manage the infrastructure.
Airflow kubernetes pod operator example software#
Google support is void installing software into an virtual environment bypassing security check.Īnd that is how we ended up with Plan C. This Again the answer is simple, security. Why stop using this option? Running dbt job in virtual environment created in Composer is causing security threats. The virtual environment is destroyed when dbt job finished. Current implementation is to create a temporary virtual environment with dbt installed for each dbt job. We can trigger the dbt DAG via pub/sub message or schedule it. The choice bypassed all the issues that Cloud Scheduler had. And depending on the version of Composer the conflicts vary. Direct installation of dbt in Composer causes failure. Why run in a virtual environment and not install as a PyPI package? The dependencies of dbt PyPI package have conflicts with Composer’s. Plan B… Composer using a virtual environment This works well if you don’t exceed the limitation of Cloud Scheduler. This works well if you don’t need any smarts in the scheduling process, such as checking that the previous load has completed before you start another load. This works well if you have a set schedule to run your dbt models but can’t trigger loads based non-dbt ingestion. This works well if you have no idea about Composer or Airflow. This was our first choice and we have a few models running using this strategy. I’d like to explain how we went about getting the Airflow using a KubernetesPodOperator choice working and also give a brief explanation as to why. We’ve been using dbt for a while now and have had a few deployment choices. Quick disclaimer: We use GCP and the solution is based on GCP only
